We use Rasa mainly to assist People Ops-related processes, and also to assist engineering.
We use Rasa Open Source edition. Note: Rasa X is free but closed source, requires either Kubernetes or Docker Compose, with 4 to 8 GB RAM.
Install Rasa on Ubuntu VM
Launch a t3a.small (2 GB) instance, with security groups default
and ssh-server-public
, with 8 GiB storage. First, run the usual Ubuntu preparation.
Note about RAM: 2 GB is required to first install rasa, 1 GB will be killed. After this, Rasa uses about 800 – 960 MiB to train, and (separately) run, so 1 GB should be enough.
Note: As of May 2021, Rasa does not support Arm/Graviton2, especially tensorflow-text:
ERROR: Could not find a version that satisfies the requirement tensorflow-text<2.4,>=2.3; sys_platform != “win32” (from rasa) (from versions: none)
ERROR: No matching distribution found for tensorflow-text<2.4,>=2.3; sys_platform != “win32” (from rasa)
Make sure .bashrc contains
export PATH=$PATH:$HOME/.local/bin
sudo apt install -y python3-dev python3-pip pipenv
pip3 install -U pip pipenv
# Use pipenv instead of plain Python3 virtual environment
mkdir rasaops
cd rasaops
pipenv install rasa
# If error here, uninstall user's virtualenv: pip uninstall virtualenv; to use globally installed virtualenv
pipenv shell
rasa init
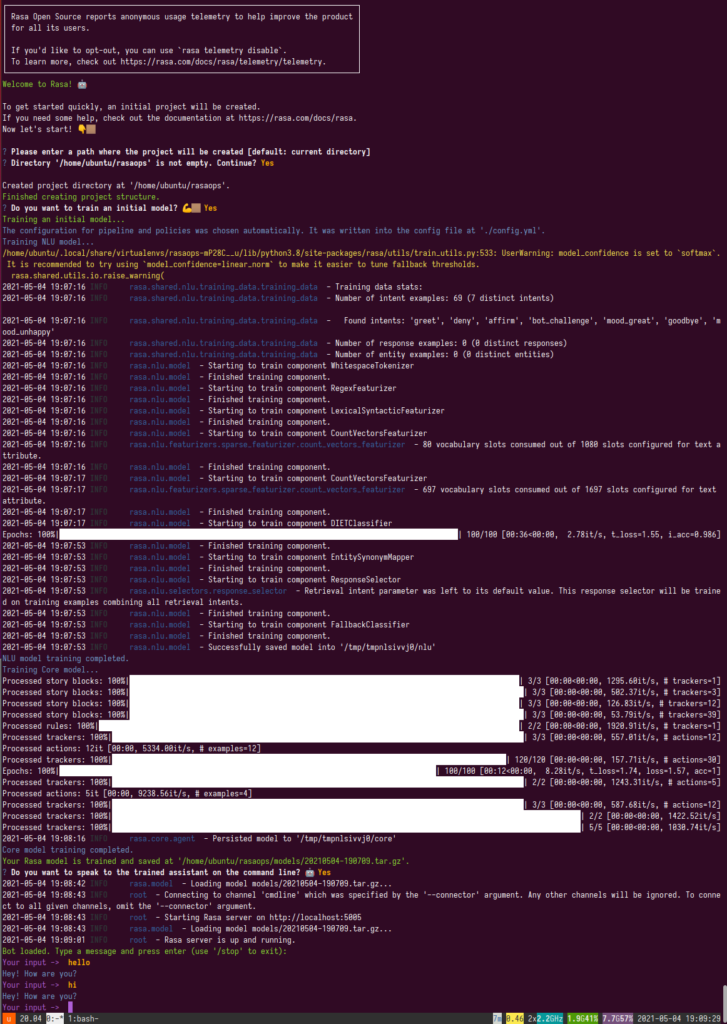
Train, Shell, and Run
After editing NLU data, you must train first. This requires 950 MB RAM but can be bigger, and if you connect via VS Code Remote-SSH then it can grow beyond that. It’s recommended to either use 2 GB or enable 512 MB swap.
rasa train
Shell to test: (to stop/quit Rasa Shell, type /stop
)
rasa shell
# or, for more debugging:
rasa shell --debug
Run server:
rasa run
# if you need more debugging:
rasa run -v
rasa run -vv
Rasa will take some time to load model. Wait until “Rasa server is up and running” message.
For any chatbot with typical functionality you’ll need Rasa Action Server as well:
pipenv rasa run actions --debug
# Useful during development
pipenv rasa run actions --auto-reload --debug
Rocket.Chat Built-in Connector
Use ALB to serve, target instance is HTTP port 5050. Alternatively without ALB, configure security group to open port 5050.
https://rasa.com/docs/rasa/connectors/rocketchat
TODO: Rocket.Chat Custom Connector
Reference: channels/rocketchat.py, channels/slack.py
Develop / Deploy Rasa using Docker / Docker Compose
Reference:
Initialize new project in current folder
# Run as host's user ID
docker run --user 1000 -v $(pwd):/app:Z rasa/rasa:latest-full init --no-prompt
# Run shell
docker run --user 1000 -v $(pwd):/app:Z rasa/rasa:latest-full shell
TODO: Deploying Rasa Assistant to AWS Lambda Docker
TODO